Resources / The Evolution Blog
What will change in 2023 for Fraud & Cybersecurity? My 5 predictions
Michael Brooks

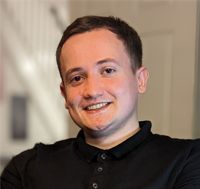
Mike has 8 years of experience in the Fraud and Cybersecurity space, specialising in delivering data-driven solutions for risk decisions across Tier 1 Fintech, Banking and eCommerce customers worldwide
I had time to reflect during the break at the end of 2022 and anticipate trends that will emerge this year. Here are my thoughts on what 2023 will bring for the Fraud & Cybersecurity industry. My experience in applied data science and product success means my predictions focus on the practicalities of how businesses collaborate with vendors to solve problems in this space.
The tl;dr
Do More with Less
- Increased cost scrutiny will justify vendor replacement and consolidation
- Niche vendor specialisms will see churn, replaced by platforms that include sufficient functionality overlap
Keep it Simple, Stupid!
- Fewer people with wider decision accountability will favour non-specialist, simpler and more manageable solutions
- New demand for orchestration and a single operating view for touchpoint solutions
Towards Decision Intelligence
- Increased automation to reduce manual processing
- Risk professionals will be refocused to manage a consortium of models combined with simple logic
Maturing of Everyday AI
- AI will be tested in attack tools, particularly for better authenticity of responses through text channels
- Personalised video deep fakes unlikely to be economical to use nefariously at scale yet
Great Expectations
- High expectations from consumers of a smooth digital experience will be the primary driver of customer retention or loss
- Risk professionals will see pressure to favour a frictionless experience instead of always reducing malicious behaviour as the priority
1. Do More with Less
With universally gloomy forecasts for 2023, budgets are being scrutinised under a harsh lens. There will be stronger justification for vendor projects which reduce operating costs:
- Like-for-like replacement of vendors that solve the same problems more cost effectively
- Consolidation of vendors; cover more use cases with the same provider
- Reducing reliance on vendors in places where ROI is weaker
- Automation of manual processes to free up employees’ time
I’ve seen vendors being managed in isolation across departments in a disjointed manner, only solving very specific problems. The pressure to cut costs will axe those that can’t prove their value wider across critical processes. If a vendor solves too niche a problem, it will be consumed by another that offers reasonable enough coverage of that domain. Demonstrating performance on an isolated problem will not be good enough to be kept around.
2. Keep it Simple, Stupid!
I’ve seen an underlying desire to simplify decisions, making them less specialist. When the budget for headcount dries up, those that remain will be expected to take wider accountability. No one wants to juggle disjointed systems and invest time to learn the specialist languages of each. Instead, there will be demand for keeping things simpler and vendor agnostic to adapt to the greater coverage and churn in personnel. A small percentage of performance drop will be seen as acceptable trade to avoid headcount or expensive vendor consulting fees.
With providers typically called by API being used in so many different places, the demand for technology that can sit as a simplification layer to link up actions across all of them will emerge. That ability fits under the category of ‘Orchestration’. Essentially this reduces down to hooking up point-in-time interactions like API calls on a platform that is available everywhere, to provide scale and consolidation of those solutions. On a technical note, the Content Delivery Network (CDN, in charge of delivering web content to the user) is a natural and great place to shift decision processes to allow that kind of scaling. Early movers will start to utilise the decision capabilities offered by both the CDNs and vendors that can deploy through them.
3. Towards Decision Intelligence
Modelling and machine learning are nothing new, but most businesses are a long way from ideal states. Models are getting into production. But as pressure increases to do more with fewer resources, it will necessitate fully embracing a rigour for machine learning as part of Business as Usual.
The two main bottlenecks here are: availability of feedback data and having employees with the right skillsets to supervise sustainable, rigourous modelling. Businesses need to fully adopt a proper feedback cycle, where cases are properly marked to make sure there is good quality feedback data to train models. The absence of feedback data could drive demand for investment in unsupervised approaches, but I've not seen these approaches return insights that are practically useful as training models directly on confirmed examples of the bad stuff.
The rigour I mentioned includes stability, continuous performance monitoring and strict versioning, even down to the granularity of the individual features that feed every model. Models and decision frameworks should be evaluated continuously (ideally daily), with alternatives available to be readily deployed when production ones drift. There is no revolutionary thinking here; the reasons for doing so will be understood by most data scientists. I’ve just not always seen the approach prioritised in reality, with busy developers in legacy and complex stacks. It tends to be seen as the ‘nice-to-have’ after the more pressing matter of simply making sure models execute decisions properly in the first place.
As reliance on models increases, risk professionals will be automated and abstracted away from very manual processes like targeted rules. Instead they will control a consortium of evolving models to pick between. The emerging field of Decision Intelligence successfully captures the aspect of effective decision practices, making sure the process is continuous, sustainable, and aligned with ideal outcomes as often as possible.
4. Maturing of Everyday AI
The use of AI for fraud has been called out as a risk for several years now, without quite coming to fruition on a large scale. I do think there will be a watershed moment this year where tooling that falls under the domain of AI is weaponised. The metaphor for how it will play out will be like plugging holes in a faulty dam. Legacy systems will be hot-fixed and patched inefficienciently to try and prevent spates of these attacks, opening new exploits in the process.
My main worry here is the skillset and awareness of how these attacks can manifest and how to defend against them, is not common knowledge. People in charge of these systems may not be equipped to defend against them. It will be a trial by fire. And as the effectiveness of attacks involving AI is proven out, so too will their frequency…
To offer balance, I don’t think highly personalised attacks will be used as widely as forecast this year (think Social Engineering via a deep fake video). The economics don’t make sense yet. It will more likely follow a path of least resistance in a similar pattern to that of email scams. Fraudsters will send out AI generated content to arrive in people’s inboxes, instant messages or through chat agents and customer service touchpoints, then capitalise and engage with people that demonstrate vulnerability to them.
Those in charge of high-net-worth individuals or text-based customer interaction points should be wary and clear on what potential destructive actions can be performed through these channels. And everyone can expect an increased barrage of text-based scams and for them to even provide more authentic responses when queried for authenticity. But I don’t think we’re at the ‘deep fake video of a loved one’ social engineering dystopia painted by many predictions. But remember, we’re only talking 2023 here… the trend for AI fraud tools is, concerningly, in the direction of better, cheaper…
5. Great Expectations
Finally, risk processes are still under continual pressure of ever-increasing demand for users to do what they need to do. Risk professionals are sometimes blind to the objective of providing the best possible user experience. Their objectives often act in direct opposition to product managers who want to incentivise growth and adoption at all costs.
But if you ask anyone, including yourself, if they’ve ever been frustrated by a digital process, the answer will undoubtedly be yes. If you analyse what has caused the frustration the number one reason would be a broken process. But a close second would be excessive friction. As new players come to the market with simplified and streamlined processes, it puts pressure on all incumbents to match expectations of amazing user experience.
An interesting corollary: we’re in a place where users actually expect to be monitored on the sites they interact with. They will claim ‘for goodness sake, why are they asking me to authenticate again, don’t they know it's me!’.. or ‘how did they let those payments that weren’t me through, I never do that!' That doesn't happen by magic. If you are in charge of decisions like these and don’t have a system that allows per-user pattern comparison in real-time, you really really need to. It would be borderline negligent to not have that capability nowadays.
On a final positive note, risk personalisation provides an opportunity to build tailored experiences. Users can be offered targeted suggestions according to what they evidence to like or dislike. Friction can be removed through passive trust indicators to improve conversion. A product or service can be designed to adapt to how a user thinks and acts. The opportunity to delight is there for the taking. Product managers with enough creativity and customer empathy to justify the developer time should look to take advantage of this in 2023.